In my 2023 Outlook (see pg. 21 of this report ), I predicted that Generative AI would take center stage in venture capital interest following the scandals and general implosion of crypto markets in 2022. Indeed, over the past twelve months, this prediction has held true, despite challenges such as reduced capital availability, a decline in deal-making activity, and a complex backdrop of rising interest rates, bank failures, and geopolitical tensions. While the Fed’s latest meeting provided cheer to the markets, I don’t believe we are completely out of the woods yet.
In today’s post I’m going to talk through some areas where I expect to see meaningful traction and investment activity in the next six to twelve months. My focus is broadly around generative AI, but more specifically on Large Language Models (LLMs). I will cover five trends gaining traction, point at interesting players within each, and why as an investor, customer, or potential employee, you should pay attention to them. In a follow up to this post, I will talk about value creation and value capture around generative AI. Click here to subscribe to InfraReadso you don’t miss future updates.
Emergence of Small Language Models (SLM) and Vertical-specific LLMs (VsLLM)
It has been conventional wisdom that bigger is better in the world of LLMs. From GPT-4 to Llama 2 to Mistral 8x7B, it is entirely expected that the most ambitious AI leaders will continue beefing up their flagship models with ever more parameters. Consider the evolutionary tree of LLMs as laid out in the April 2023 paper Harnessing the Power of LLMs in Practice: A Survey on ChatGPT and Beyond –
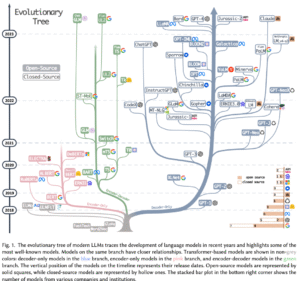
Despite the parameters-focused arms race in this initial phase, I believe, the more compelling and capable models over time are likely to emerge from focused data sets that are use-case-, language- or geography-specific (or some combination of these). These smaller models don’t have to be independent of the general-purpose LLMs. I fully expect a large number of the SLMs and VsLLMs to be built on the work that has already gone into existing, general-purpose models.
Some examples from the recent past and on the horizon are –
- BloombergGPT, a finance focused model trained on Bloomberg’s formidable finance-specific data resources
- Noetica, a New York based firm that just got funded by Lightspeed and has built a platform for evaluating capital market deal terms during negotiation
- Krutrim, and Sarvam, both focused on building LLMs for Indic languages
- Flip AI, which has trained its LLM on operational data from complex infrastructure environments and claims to supercharge observability workflows with its proprietary technology
- Dozens of derivative, task-specific models built on top of open-source models like Llama and Mistral. Here are examples for coding, math, multi-modal tasking, reasoning, chatbots and for running on entry-level hardware
Multi-modality, Context-Awareness, and Longer Outputs: Going Beyond Chat
My initial excitement on viewing Google’s Gemini launch video was tempered on learning that it had been editedto look smoother than it actually was. Despite the revelation, I think the video paints a picture of how multi-modality in LLMs turbocharges human-computer interaction. I expect multi-modality (audio and visual processing for instruction and inference) to be table stakes for all existing and future large language models that want to be relevant for general-purpose use cases.
The “very” cool Gemini Launch video
This does not mean specialized models for specific use cases like spoken language, music, image, and video generation will cease to be relevant. All of those will continue improving in parallel, at least in the near-future.
ChatGPT’s success has anchored chat as the primary use case for leveraging LLMs. I think that’s will change fast. Chat is quite limited when seen from the context of enterprise. Most modern enterprise workflows are complex interactions that require large context windows, multiple threads, and the ability to generate coherent and consistent long-form, multi-modal content. Today’s crop of large language models were not designed for most enterprise use cases except, perhaps customer service and programming. Rapidly dating knowledge snapshots are a limitation as well.
Recent approaches to overcome these limitations include Retrieval-Augmented Generation (RAG) coupled with frameworks like LangChain, LlamaIndex and AutoGen, but have a long way to go. OpenAI’s DevDay announcements were specifically targeted at many of these challenges and set the stage for competitors like Anthropic, Cohere, Falcon, Gemini, Llama and Mistralto follow and catch up.
Evolving Architectures: Beyond Bigger, Towards Smarter
If you frequent the AI and Machine Learning corners of Twitter, GitHub YouTube, or Hugging Face, you’re likely familiar with arXiv. arXiv serves as an archive of ground-breaking research in several technical fields, with one of the most prominent areas being computer science. The pace of new ideas being posted on arXiv, and then implemented into working prototypes around all axes of generative AI is simply breathtaking.
Fueled by capital and compute resources from VCs, research labs, and large-tech, researchers are pushing the boundaries of LLM capabilities while also reducing compute overhead. Efficient and sustainable inference times are a prime target, and recent advancements reveal remarkable strides in running models with significantly fewer resources. This ties closely to the trend of domain-specific models mentioned previously. Some recent examples highlighting this trend are –
- Mistral AI’s Mixtral 8x7B, a powerful large language model with a unique architecture known as a sparse mixture-of-experts network. The model has 8 distinct sets of parameters, each acting as an “expert” in different aspects of language processing. While having a large total parameter count (46.7B), Mixtral only uses a smaller portion (12.9B) per token thanks to expert selection. This means that processing a token takes the same time and resources as a smaller 12.9B model, despite the larger overall parameter size.
- Microsoft’s Phi-2leverages specially curated ‘textbook quality data’ to excel on complex benchmarks, outperforming even models with up to 25 times the number of parameters. Its performance is further boosted by building upon the work done with its predecessor, Phi-1.5.
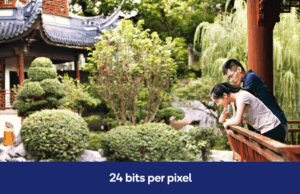
- Quantization is an accessible technique for reducing model size, and therefore memory requirements and inference times. Simply put, quantization involves using lower precision weights to shrink the amount of memory required to load and run the model. The tradeoff is lower accuracy but quantization seems to work for many use cases. Click here for a good overview of the technique and some applications.
Security, Governance and Compliance
Remember Tay? Released in the wild (i.e. Twitter) in May 2016 by Microsoft researchers, Tay was meant to be “a social and cultural experiment” that actually ended up reflecting what a cesspool Twitter could be. Unfortunately, Tay is not the only example of missteps in generative AI by large tech. Meta launched Galactica two weeks before ChatGPT made its debut, and abruptly shut it down shortly after. Galactica was intended to help scientists and other users “summarize academic papers, solve math problems, generate Wiki articles, write scientific code, annotate molecules and proteins, and more.” Doesn’t sound all that different from what ChatGPT offered shortly after, albeit to a far different reception. The dismissive tone of this MIT Technology Review article, written weeks before ChatGPT was released publicly, gives one a sense of how incredibly well OpenAI managed the launch compared to everything that came before it.
Large Language Models are complex and the technology behind them is evolving rapidly. The fact is that we are still trying to fully understand why and how they really work. Researchers have been busy trying to hack, crack and compromise models across the board. AI researcher and educator Andrej Karpathy gives a quick overview of some techniques including prompt injection, jailbreaking, and data poisoning in his hour-long intro to LLMs (which I recommend.)
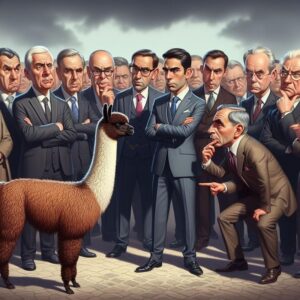
Against this checkered history, and given the world-changing potential of generative AI, it is not surprising that companies and governments are starting to draft regulations around the technology. I expect to hear a lot more on actions by bad actors and efforts to curb them in the coming year. Below are some of the key initiatives and privacy+security related startups I’m paying attention to –
- Executive Order on the Safe, Secure, and Trustworthy Development and Use of Artificial Intelligence issued by President Biden in late October and two summaries – E&Y, epic.org
- The European Union’s Artificial Intelligence Act – good summary here; related post – John Loeber discusses why Europe needs to start creating stuff rather than freeloading American (and Asian?) ingenuity
- Meta’s Purple Llama – “an umbrella project featuring open trust and safety tools and evaluations to help developers build responsibly with AI models”
- LLM data security startups Lakera, Deepchecks and Deasie, all of whom have recently raised seed rounds
- Privacy and compliance focused startups Harmonic Security, Citrusx and DynamoFL
Climate and Sustainability
From a recent episode of This American Life, I learned that the Paris Agreement commits the US to a 50% emissions reduction by 2030, relative to 2005. The good news is that we are already halfway there, while the remaining 25% seems achievable. Rather, it seems achievable if we do not factor in the impact of generative AI on world energy consumption.
Generative AI is extremely power-hungry. An article in the Wall Street Journal claims “global electricity consumption for AI systems could soon require adding the equivalent of a small country’s worth of power generation to our planet.”I’m not a climate scientist, but this does not sound great for the planet.
The need for additional power in the near future is not a hypothetical. Microsoft is already planning to power generative AI using nuclear power in the near future. The company is partnering with Constellation Energy, which projects “new demand for power for data centers could be five or six times what will be needed for charging electric vehicles.”
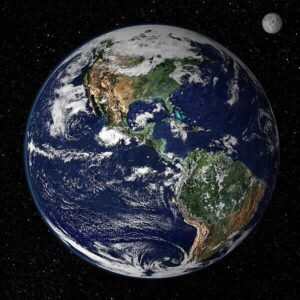
If climate change is the planet’s most existential crisis, and generative AI drives us closer to an abyss from which there is no turning back, should we pump the brakes? Or perhaps use nuclear fusion – a technology that is literally being invented as we speak? How about redesigning compute and storage from the ground up for AI-specific tasks like d-Matrix and Cerebras? Or using opitcs like Lightmatter and Ayar Labs? Alternatively, we could focus our collective energies on making quantum computingreal and present.
I have a feeling the right answer is a combination of all of the above. Figuring out how to solve for the externalities of generative AI should be on top of the priority list of every deep-tech investor, from Silicon Valley to Shenzhen.
That brings me to the end of my prognostication – hope you enjoyed reading it and found some ideas to mull over. I’d love to hear your thoughts and comments. Please feel free to add them below or reach out directly.
I plan to follow up with a short review of existing business models around generative AI and how I expect them to evolve in the near future. Subscribe to the newsletter hereto make sure you don’t miss it.